KI für die klinische Praxis fit machen
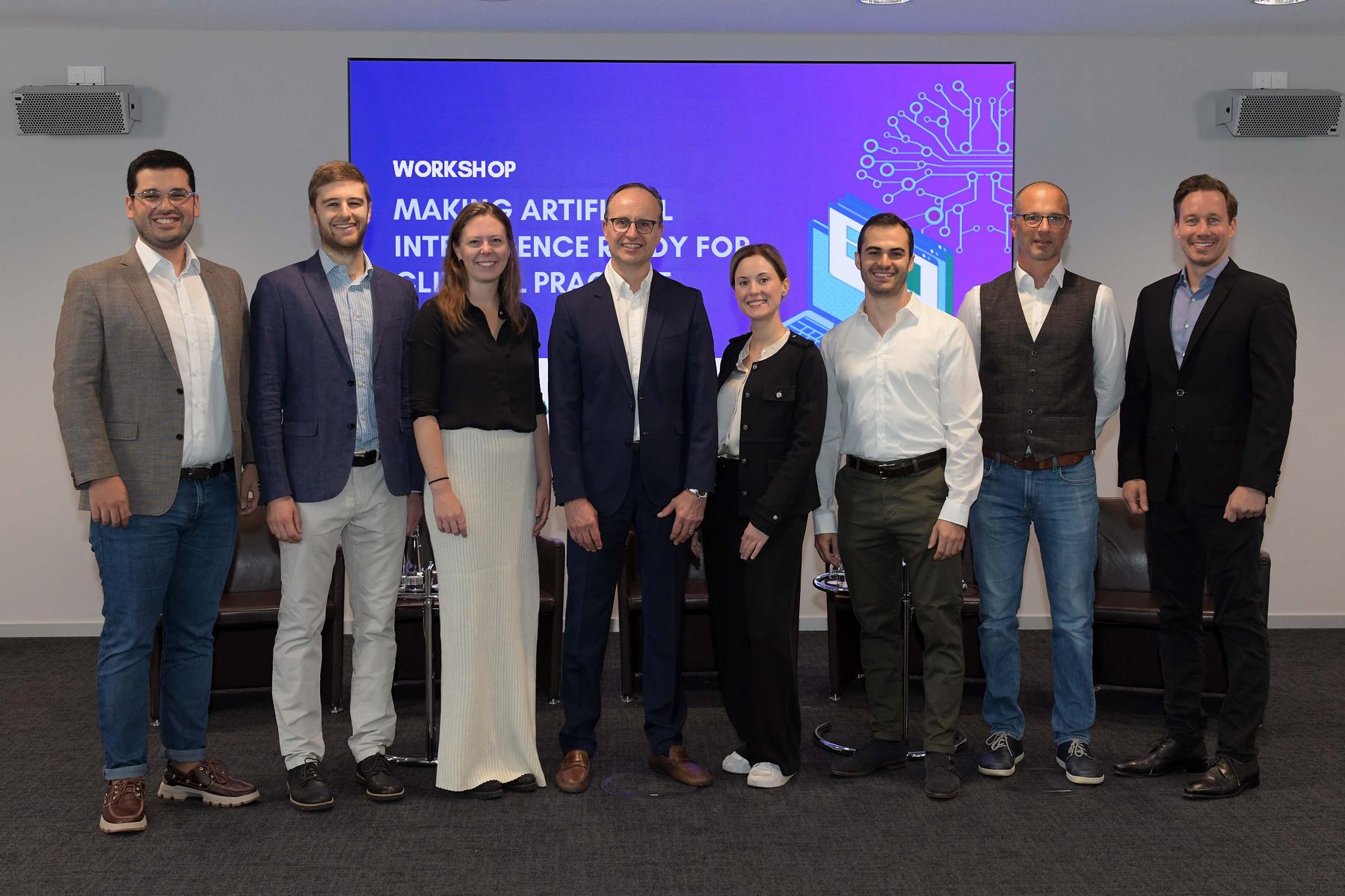
Wichtige Themen zusammengefasst
Nach der Eröffnungsrede von Prof. Dr. Lars French präsentierte PD Dr. Tobias Lasser die neuesten Erkenntnisse und Entwicklungen seiner TUM-Forschungsgruppe "Computational Imaging and Inverse Problems" im Kontext der klinischen Entscheidungsunterstützung in der Radiologie und Dermatologie. Während KI-Algorithmen bereits in bestimmten Anwendungsszenarien – wie der Erkennung von Lungenerkrankungen auf Röntgenaufnahmen oder Hautkrankheiten auf Fotografien – hervorragende Leistungen erbringen, fehlen noch einige Elemente für die routinemäßige klinische Anwendung.
Ein Beispiel sind neuartige Fusionsverfahren, welche Bilder mit zusätzlichen Patientendaten für eine verbesserte diagnostische Qualität verschmelzen. Ein weiteres Beispiel ist der Beweis, dass winzige Bilddetails, die routinemäßig verworfen werden, eine hohe Relevanz im Hinblick auf Algorithmusresultate besitzen. Ein anderes Beispiel bezieht sich auf die Interpretierbarkeit der Ergebnisse der Algorithmen, damit Ärzte deren klinischen bzw. Einsatz in der Praxis besser verstehen und umsetzen können. Zu guter Letzt können Patientendaten ausgeschlossen werden, für welche die Algorithmen – aufgrund von Beschränkungen im Trainingsszenario – keine genaue Vorhersage treffen können. Alessandro Wollek, Doktorand in Dr. Lassers Forschungsgruppe, erläuterte einige dieser neuartigen Erkenntnisse in seiner Präsentation.
Im Anschluss stellte Dr. Sebastian Krammer die Forschungsstudien der Gruppe zur Erkennung von Hauterkrankungen mittels Bildanalyse vor. Er betonte die Digitalisierungsfortschritte anhand der sogenannten Software Avelios, welche die Dokumentationseffizienz erheblich gesteigert hatte. Diese strukturierte Dokumentation ermöglicht bis zu 2.000 Datenpunkte im Falle einer einzigen Patientenbehandlung. Mit diesen zusätzlichen Metainformationen wird derzeit ein neuer Algorithmus zur Erkennung von Hauterkrankungen entwickelt, der auf einem multimodalen, mehrstufigen Datenintegrationsprozess basiert.
Prof. Dr. Michael Ingrisch, Leiter der Clinical Data Science Group am LMU Klinikum, ist davon überzeugt, dass es entscheidend ist, die richtigen Fragen zu stellen – klinische Fragen mit klinischer Relevanz. Wichtig sei vor allem, dass diese Fragen im klinischen Kontext beantwortet werden, beispielsweise durch den Einsatz von KI-Systemen auf kritische Untergruppen bezogen. PD Dr. Bastian Sabel erläuterte in seinem Vortrag die schrittweise Implementierung der KI in der Radiologie. Trotz der Vorteile dieser Technologie betonte er die Notwendigkeit, ethische und praktische Fragen anzugehen, besonders in Bezug auf Datenschutz und die Rolle des menschlichen Fachwissens in einer immer digitaleren Medizinwelt.
Das TUM Embedded Ethics Team von Prof. Dr. Alena Buyx berichtete über die Einbettung der Ethik innerhalb der Forschungskooperation. Momentan wird Ethik oft als ein nachträglicher Gedanke verstanden. Das Team hat es sich zur Aufgabe gemacht, Ethik "an die Werkbank" zu bringen und ethische Fragen dann zu diskutieren, wenn sie im Projekt auftreten. Im Rahmen des DR-AI-Projekts hatte das Team seinen Ansatz mit Hilfe einer Interviewstudie umgesetzt und ethonografische Feldforschung betrieben. Neben Einblicken in die biomedizinische Ethikforschung ermöglichte der Forschungsauftrag des Teams auch, den Projektansatz besser an die Bedürfnisse von Informatikern und Klinikern anzupassen.
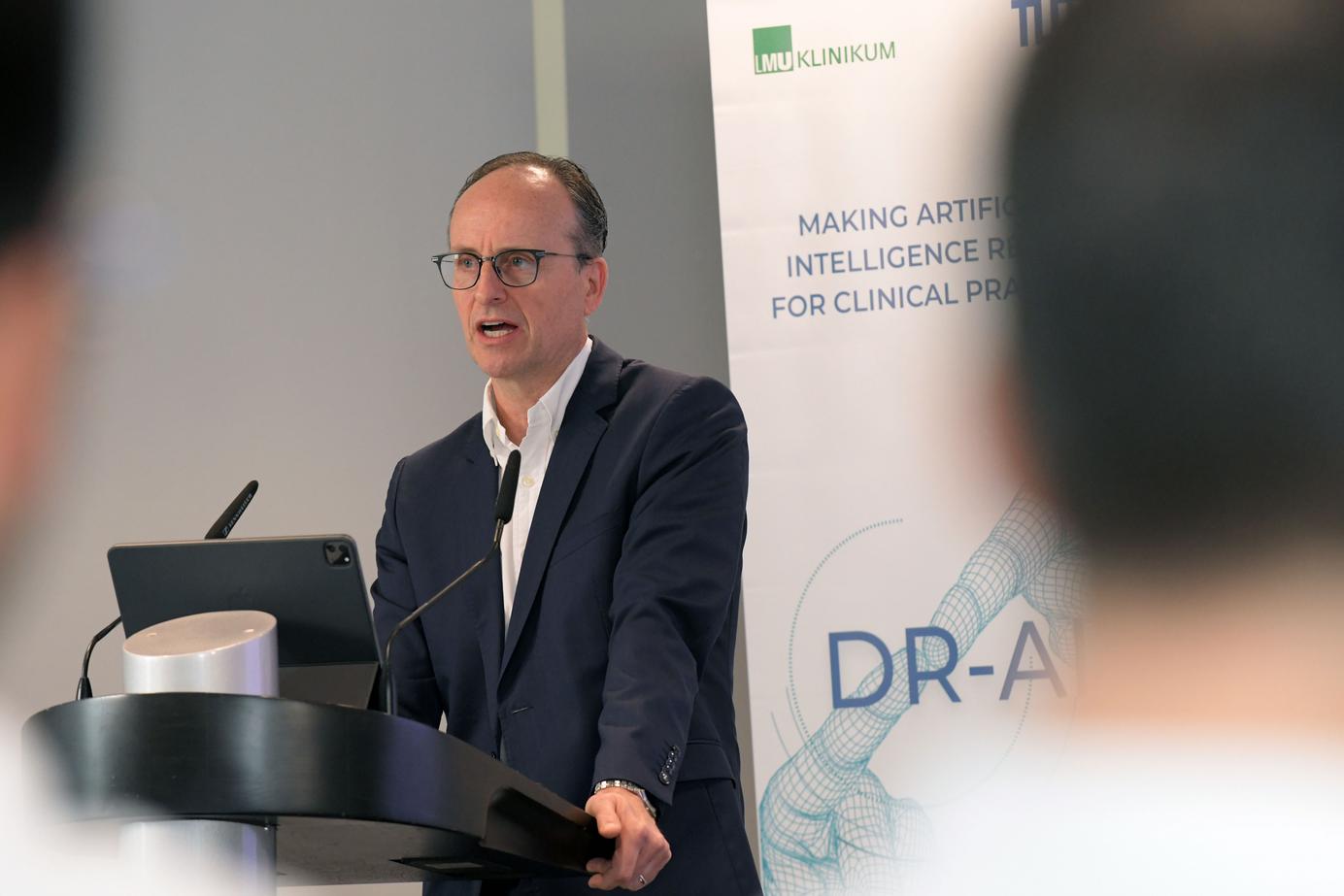
Abschließend diskutierten die Kooperationspartner in einer Podiumsdiskussion den aktuellen Forschungsstand, welcher Anreize für gemeinsame Lösungsansätze bot. Unumstritten war, dass es einer gut ausgebauten digitalen Infrastruktur bedarf; auch ein Mangel an strukturierten Daten wurde verzeichnet.
„Zusammenfassend lässt sich sagen, dass dieser Workshop sowohl den Teilnehmenden als auch den Rednerinnen und Rednern einen produktiven Tag des Lernens und des Ideenaustauschs ermöglichte“, sagte Prof. Dr. Lars French, Leiter der DR-AI-Arbeitsgruppe. Die Workshoperfolge, Erkenntnisse und gestärkte Partnerschaften werden die DR-AI Forschungsgruppe auch in Zukunft befähigen, die Herausforderungen der Künstlichen Intelligenz gesamtheitlich und nachhaltig zu lösen.
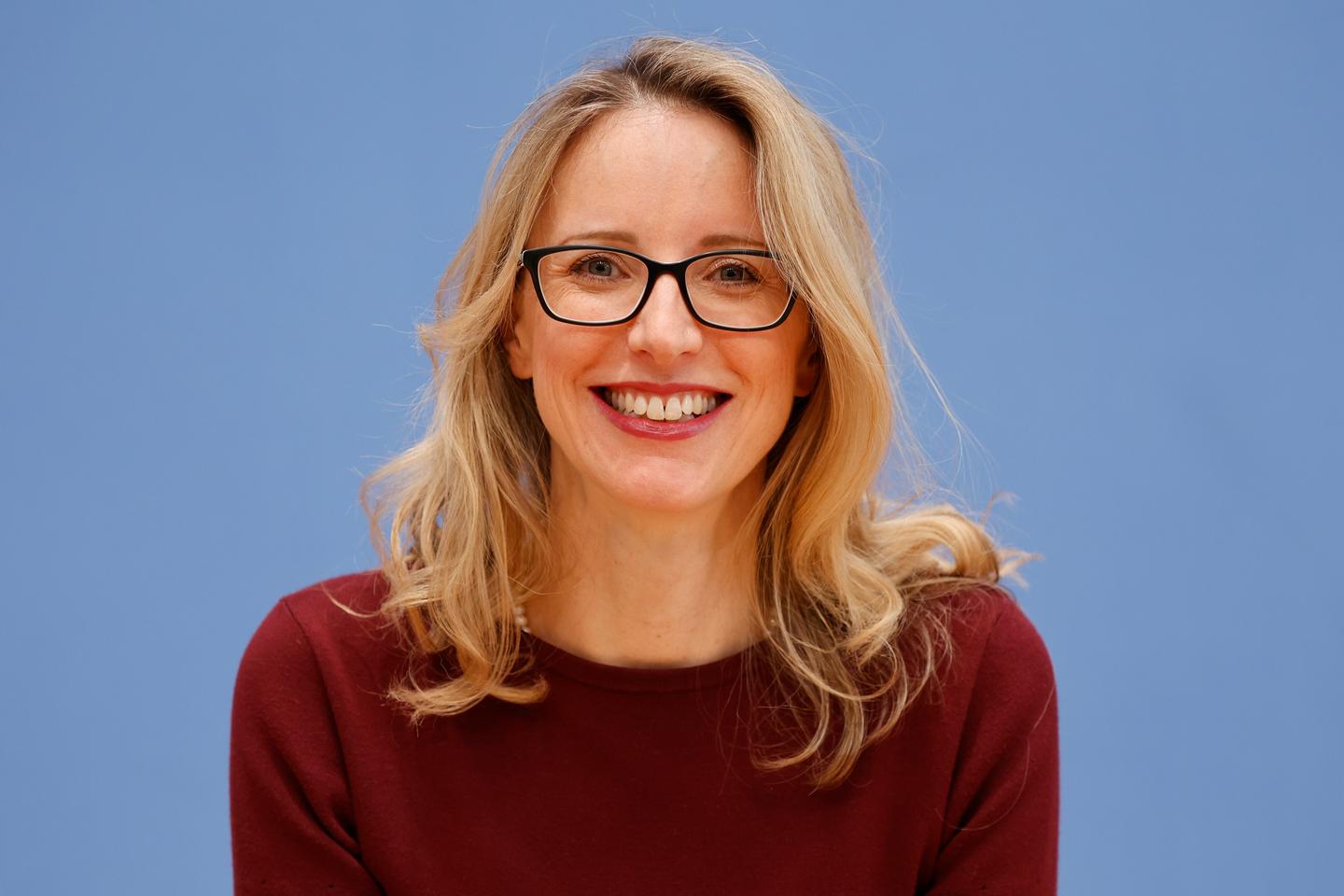
"Die DR-AI-Workshops haben gezeigt, wie wichtig es ist, Forschungsgruppen von Klinikärztinnen und -ärzten, Computer Spezialisten und Ethiker im Auftrag mordernster KI für den Gesundheitsbereich zusammen zu bringen. Die Workshops offerierten somit eine Basis, welche es gestattete unsere Forschung zu präsentieren – viel wichtiger aber noch unsere Ideen kritisch zu „challengen“. Die Diskussionen verhalfen unserer fachübergreifenden Arbeit zu einem Durchbruch, denn so konnten wir am besten identifzieren, welche Dermatologie und Radiologie bezogenen Algorithmen notwendig sind, um den höchsten ethischen Standards gerecht zu werden. Wir freuen uns schon jetzt auf unseren Austausch im Zusammenhang mit neuen Projekten, damit wir unsere Klinik und Universität übergreifenden Initiativen wie diejenige von DR-AI erfolgreich weiterverfolgen."
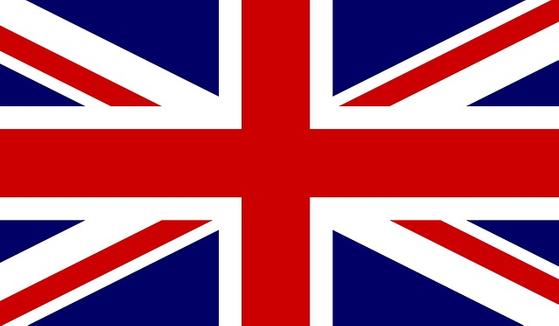
Making AI ready for clinical practice
Scientists from various Germany and EU based AI working groups actively engaged in scientific dialogue with one another in Munich
The DR-AI Research Consortium presented its subprojects and state-of-the-art findings in the fields of artificial intelligence in dermatology and radiology vis-à-vis a hybrid audience in Munich on September 1st, 2023. DR-AI reflects on a research collaboration between LMU Dermatology and LMU Radiology as well as TUM Informatics and TUM Medical Ethics devoted to artificial intelligence applications for medical purposes. The German Federal Ministry of Health supported this groundbreaking consortium with €2.4 million. It is led by Prof. Lars French, Director of the Clinic and Polyclinic for Dermatology and Allergy at LMU University Hospital Munich.
Summarized key events
Prof. Lars French's opening speech was followed by PD Dr. Tobias Lasser presentation on the latest findings and developments of his TUM research group “Computational Imaging and Inverse Problems” in the context of clinical decision support in radiology and dermatology respectively.
While AI algorithms already excel in correctly identifying diseases in certain application scenarios, such as lung diseases in X-ray radiographs or skin diseases in photographs, several elements for daily clinical usage are still missing representing the focus of Dr. Lasser’s research group.
One example are novel fusion schemes merging images with additional patient information for better diagnostic quality. Another example was the proof that tiny image details that are routinely discarded have – not surprisingly - high relevance on algorithms’ outcomes. Yet another example is related to the interpretability of the algorithms’ outcomes, so that doctors better understand how to apply algorithms in clinical practice, thereby building trust and providing support. Last but not least patient data can be excluded for which algorithms cannot make an accurate prediction due to limitations in training data set.
Alessandro Wollek, a PhD student of Dr. Lasser’s research group, presented several of those novel findings in detail during his presentation.
Dr. Sebastian Krammer’s presentation outlined the group's research studies on detection of skin diseases using image analysis. Stressing how digitization with so-called software Avelios contributed to documentation efficiency at LMU University Hospital, Dr. Krammer exemplified up to 2,000 structured data points in case of a single patient treatment. Based on this meta data abundance, a new algorithm for detecting skin diseases has been developed characterized by a multimodal, multistep data integration process.
Prof. Michael Ingrisch, Director of the LMU Clinical Data Science Group, is convinced that asking the right questions - clinical questions with clinical relevance - is crucial. Above all, it would be important that these questions are answered in a clinical context, e.g. by using AI systems on critical subgroups.
PD. Dr. Bastian Sabel discussed gradual implementation of AI in radiology, i.e. phase by phase, in his lecture. Despite the advantages of this technology, he emphasized the need to address ethical and practical concerns, especially regarding data privacy and the role of human expertise in an increasingly digital medical landscape.
Prof. Alena Buyx's TUM Embedded Ethics team reported on how they embedded ethics within the consortium’s project setting. As of now, “ethics” is often perceived as an afterthought. Therefore, the team’s mission is to bring ethics “to the workbench”, e.g. by discussing ethical issues as soon as they arise.
In the context of the DR-AI project, the aforementioned approach was implemented by conducting an interview study with all participants and carrying out ethnographic fieldwork. The study’s results stress researcher’s responsibility in machine learning development processes and, contribute to high-quality decision-making in medicine.
Besides offering insights into biomedical ethics research, the team’s work package provided an early example of successful embedded ethics application and yielded essentials tailoring the approach closer to computer scientists’and clinicians’needs.
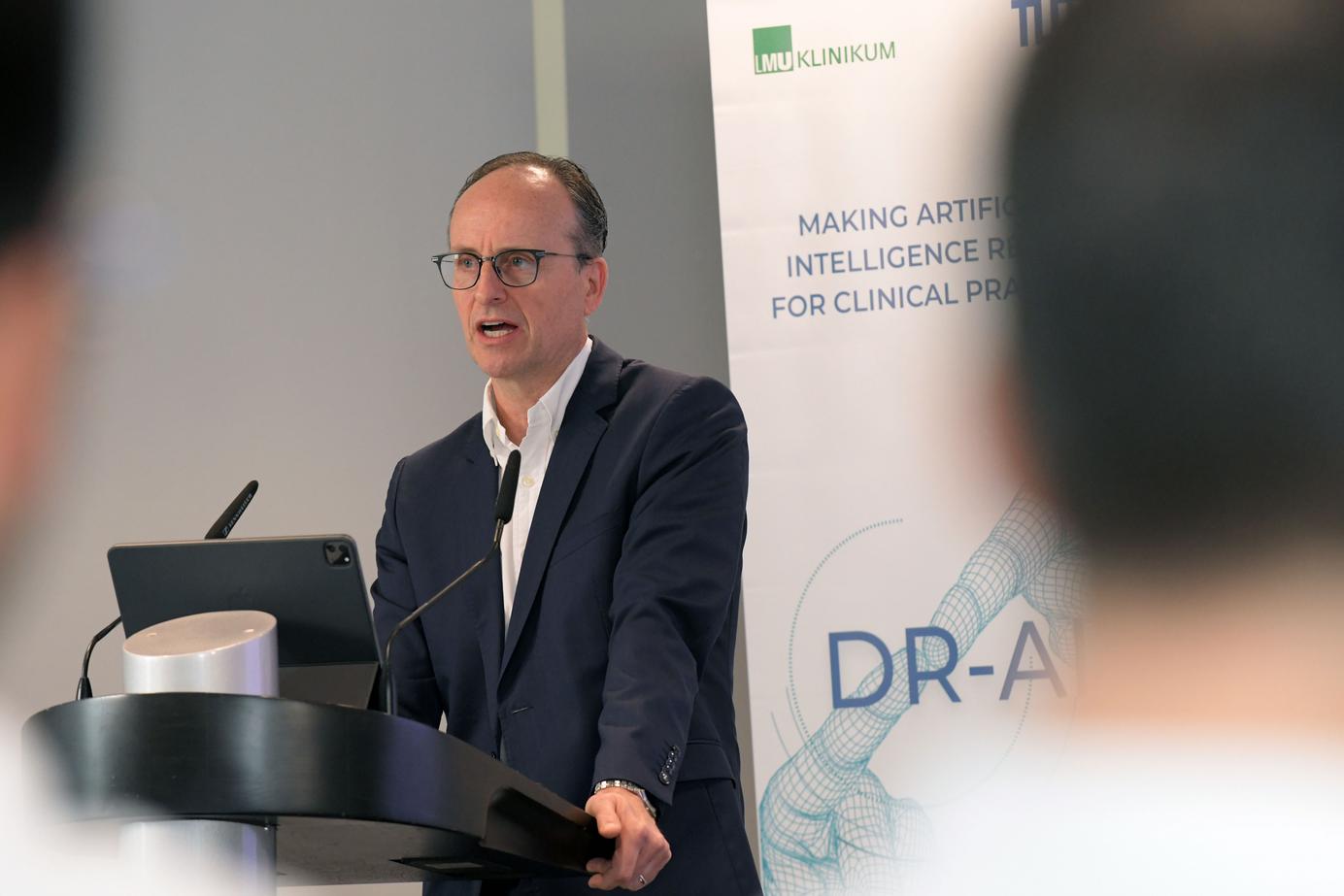
The DR-AI Research Consortium concluded its workshop with a panel discussion allowing for discussion as well as providing a shared problem-solving stimulus. In the discussion, the successful translation of state-of-the-art machine learning algorithms to clinical practice was unanimously identified as a continuous challenge. Further, the need for a well-developed digital infrastructure as development environment and the lack of structured data were highlighted.
“All in all, this workshop created the space for a fruitful and productive day of learning and sharing ideas among all attendees and speakers”, said Prof. Lars French, head of the DR-AI Working Group. Building on its workshop success, findings and strong partnership ties within the AI research community, the DR-AI Research Consortium members will continue to comprehensively and sustainably tackle AI challenges in the future.
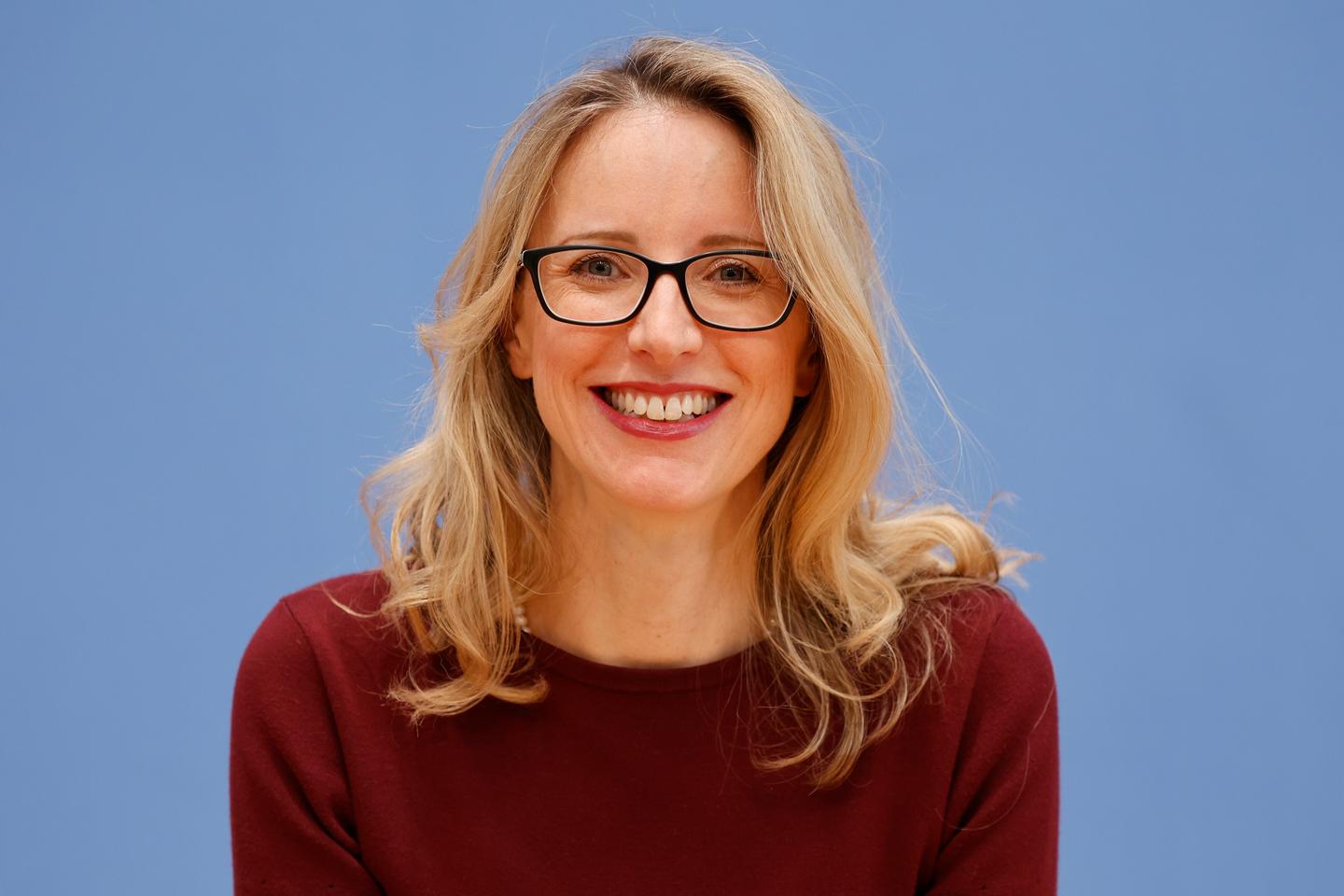
"The DR-AI workshops have demonstrated how fruitful it is to bring together a consortium of clinicians, computer scientists, and ethicists to develop state-of-the-art machine learning healthcare research. The workshops served as a shared space where we could present our findings but, most importantly, go off each other’s ideas. The discussions leveraged the potential of interdisciplinary working and gave us new insights into what is needed to create dermatological and radiological algorithms corresponding to the highest ethical standards. We look forward to continuing our discussions in new projects and hope for the successful continuation of cross-clinic and cross-university initiatives like DR-AI."